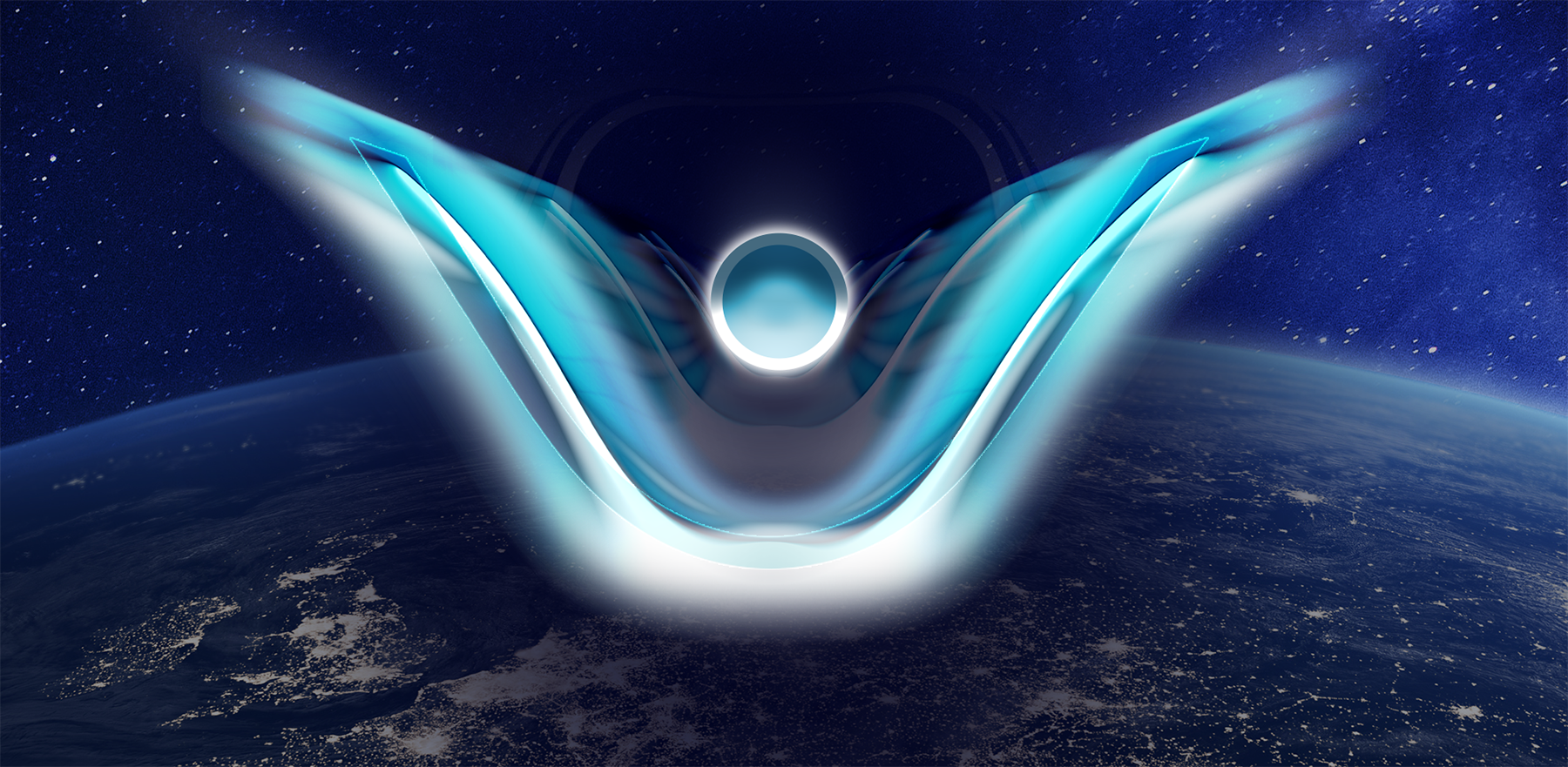
The Concept of Virtual Identity
The concept of virtual identity refers to the way individuals and entities present themselves in digital environments. It encompasses aspects such as online profiles, avatars, digital footprints, and personal data. Virtual identity has become an integral part of modern life, as more and more people interact with each other and with organizations through digital channels. However, virtual identity also raises significant ethical, legal, and technological challenges that need to be addressed to ensure its responsible and beneficial use.
=== Historical Overview of Virtual Identity Systems
Virtual identity systems have been around for decades, dating back to the early days of the internet when bulletin board systems (BBS) and multi-user dungeons (MUD) allowed users to create online personas. The advent of social media platforms such as Facebook, Twitter, and Instagram in the 2000s gave rise to a new era of virtual identity, where millions of users could build and maintain online profiles that reflected their real-life identities. More recently, blockchain-based identity systems are being developed as a way to provide decentralized and secure virtual identity management.
=== Types of Virtual Identity Systems
There are several types of virtual identity systems, each with its own characteristics and use cases. Some examples include:
- Personal identity systems: These are systems that allow individuals to create and manage their digital identities, such as social media profiles, email accounts, and online banking accounts.
- Organizational identity systems: These are systems that allow organizations to establish their digital identities, such as corporate websites, online stores, and customer relationship management (CRM) platforms.
- Federated identity systems: These are systems that allow users to access multiple digital services using a single set of credentials, such as the OpenID Connect protocol.
- Self-sovereign identity systems: These are systems that give individuals full control over their digital identities, including the ability to manage their personal data, share it with others, and revoke access when needed.
=== Ethics of Virtual Identity Creation and Use
The creation and use of virtual identity raise numerous ethical concerns that need to be addressed. For instance, virtual identity systems can perpetuate bias, discrimination, and exclusion if they are designed or used in ways that favor certain groups over others. Furthermore, virtual identity systems can compromise individual privacy and autonomy if they collect and store personal data without consent or use it for nefarious purposes. Ethical considerations should be central to the design, deployment, and management of virtual identity systems to ensure that they serve the public good.
=== Regulating Virtual Identity: Legal Frameworks
Virtual identity systems are subject to various legal frameworks that govern their creation and use. These frameworks include data protection regulations, privacy laws, consumer protection laws, and intellectual property laws. For example, the General Data Protection Regulation (GDPR) in Europe imposes strict requirements on the processing of personal data, including the right to be forgotten, the right to access, and the right to rectification. Legal frameworks can help mitigate the risks associated with virtual identity systems and provide a framework for ethical and responsible use.
=== Case Study: Virtual Identity in Social Media
Social media platforms have become a major source of virtual identity for millions of people worldwide. Users can create online profiles that include personal information, photos, videos, and posts. These profiles can be used to connect with friends and family, share opinions and experiences, and engage with content from others. However, social media platforms have also been criticized for their handling of user data, their role in spreading misinformation and hate speech, and their impact on mental health and well-being. Social media companies are facing increasing pressure to adopt more responsible and transparent practices that protect users’ privacy and mitigate harm.
=== Virtual Identity and Artificial Intelligence
Artificial intelligence (AI) is playing an increasingly prominent role in virtual identity systems. AI algorithms can be used to analyze large amounts of data to identify patterns, trends, and correlations, which can be used to improve virtual identity management. For example, AI can be used to detect fraudulent activities, prevent identity theft, and personalize user experiences. However, AI also raises significant ethical concerns, such as bias, discrimination, and lack of transparency. Virtual identity systems that rely on AI should be designed and implemented in ways that prioritize ethical considerations and ensure that the benefits outweigh the risks.
=== Benefits of Virtual Identity Systems
Virtual identity systems offer numerous benefits to individuals, organizations, and society as a whole. Some of these benefits include:
- Improved access to digital services and resources
- Enhanced personalization and customization of user experiences
- Increased efficiency and convenience in digital transactions
- Better security and fraud prevention
- Greater transparency and accountability in identity management
Virtual identity systems can also facilitate social inclusion and empowerment by providing individuals with a platform to express their identity, connect with others, and participate in public discourse.
=== Risks and Challenges of Virtual Identity
Virtual identity systems also pose significant risks and challenges that need to be addressed. Some of these risks include:
- Privacy violations and data breaches
- Identity theft and fraud
- Discrimination and bias
- Cyberbullying and online harassment
- Misinformation and propaganda
Virtual identity systems can also exacerbate existing social and economic inequalities and widen the digital divide if they are not designed and implemented in inclusive and equitable ways.
=== The Future of Virtual Identity: Trends and Projections
The future of virtual identity is likely to be shaped by several trends and projections. These include:
- Increasing adoption of blockchain-based identity systems
- Greater focus on privacy and data protection
- Advancements in AI and machine learning
- Growing demand for self-sovereign identity management
- Emphasis on inclusivity and accessibility
The future of virtual identity will also be shaped by societal, cultural, and political factors that are difficult to predict but will undoubtedly play a significant role.
The Importance of Virtual Identity
Virtual identity is a crucial aspect of modern life that offers both opportunities and challenges. As digital technologies continue to shape the way we interact and communicate with each other, virtual identity will become even more important in shaping our digital selves. To ensure that virtual identity serves the public good and respects individual rights and freedoms, it is essential to adopt an ethical, legal, and responsible approach to its creation and use. By doing so, we can harness the benefits of virtual identity while mitigating its risks and challenges.
=== References and Further Reading
- Solove, D. J. (2013). Understanding privacy. Harvard University Press.
- Goffman, E. (1959). The presentation of self in everyday life. Doubleday.
- European Union. (2016). General Data Protection Regulation (GDPR). Retrieved from https://eur-lex.europa.eu/legal-content/EN/TXT/?uri=CELEX%3A32016R0679
- Kantara Initiative. (2019). Identity and Access Management for the Internet of Things (IoT) Primer. Retrieved from https://kantarainitiative.org/download/80863/
- World Economic Forum. (2018). Empowering Identity: Blockchain for Development – A Primer. Retrieved from http://www3.weforum.org/docs/WEF_Empowering_Identity_Blockchain_for_Development_2018.pdf
- World Bank Group. (2016). Digital Dividends. Retrieved from https://openknowledge.worldbank.org/bitstream/handle/10986/23347/9781464806711.pdf