An entity–relationship model (or ER model) describes interrelated things of interest in a specific domain of knowledge. A basic ER model is composed of entity types (which classify the things of interest) and specifies relationships that can exist between entities (instances of those entity types).

An entity–attribute-relationship diagram for an MMORPG using Chen’s notation.
In software engineering, an ER model is commonly formed to represent things a business needs to remember in order to perform business processes. Consequently, the ER model becomes an abstract data model, that defines a data or information structure which can be implemented in a database, typically a relational database.
Entity–relationship modeling was developed for database and design by Peter Chen and published in a 1976 paper,[1] with variants of the idea existing previously.[2] Some ER models show super and subtype entities connected by generalization-specialization relationships,[3] and an ER model can be used also in the specification of domain-specific ontologies.
Introduction
An E-R model is usually the result of systematic analysis to define and describe what is important to processes in an area of a business. It does not define the business processes; it only presents a business data schema in graphical form. It is usually drawn in a graphical form as boxes (entities) that are connected by lines (relationships) which express the associations and dependencies between entities. An ER model can also be expressed in a verbal form, for example: one building may be divided into zero or more apartments, but one apartment can only be located in one building.
Entities may be characterized not only by relationships, but also by additional properties (attributes), which include identifiers called “primary keys”. Diagrams created to represent attributes as well as entities and relationships may be called entity-attribute-relationship diagrams, rather than entity–relationship models.
An ER model is typically implemented as a database. In a simple relational database implementation, each row of a table represents one instance of an entity type, and each field in a table represents an attribute type. In a relational database a relationship between entities is implemented by storing the primary key of one entity as a pointer or “foreign key” in the table of another entity.
There is a tradition for ER/data models to be built at two or three levels of abstraction. Note that the conceptual-logical-physical hierarchy below is used in other kinds of specification, and is different from the three schema approach to software engineering.
- Conceptual data model
- This is the highest level ER model in that it contains the least granular detail but establishes the overall scope of what is to be included within the model set. The conceptual ER model normally defines master reference data entities that are commonly used by the organization. Developing an enterprise-wide conceptual ER model is useful to support documenting the data architecture for an organization.
- A conceptual ER model may be used as the foundation for one or more logical data models (see below). The purpose of the conceptual ER model is then to establish structural metadata commonality for the master data entities between the set of logical ER models. The conceptual data model may be used to form commonality relationships between ER models as a basis for data model integration.
- Logical data model
- A logical ER model does not require a conceptual ER model, especially if the scope of the logical ER model includes only the development of a distinct information system. The logical ER model contains more detail than the conceptual ER model. In addition to master data entities, operational and transactional data entities are now defined. The details of each data entity are developed and the relationships between these data entities are established. The logical ER model is however developed independently of the specific database management system into which it can be implemented.
- Physical data model
- One or more physical ER models may be developed from each logical ER model. The physical ER model is normally developed to be instantiated as a database. Therefore, each physical ER model must contain enough detail to produce a database and each physical ER model is technology dependent since each database management system is somewhat different.
- The physical model is normally instantiated in the structural metadata of a database management system as relational database objects such as database tables, database indexes such as unique key indexes, and database constraints such as a foreign key constraint or a commonality constraint. The ER model is also normally used to design modifications to the relational database objects and to maintain the structural metadata of the database.
The first stage of information system design uses these models during the requirements analysis to describe information needs or the type of information that is to be stored in a database. The data modeling technique can be used to describe any ontology (i.e. an overview and classifications of used terms and their relationships) for a certain area of interest. In the case of the design of an information system that is based on a database, the conceptual data model is, at a later stage (usually called logical design), mapped to a logical data model, such as the relational model; this in turn is mapped to a physical model during physical design. Note that sometimes, both of these phases are referred to as “physical design.”
Entity–relationship model
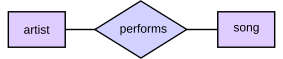
Two related entities

An entity with an attribute

A relationship with an attribute
An entity may be defined as a thing capable of an independent existence that can be uniquely identified. An entity is an abstraction from the complexities of a domain. When we speak of an entity, we normally speak of some aspect of the real world that can be distinguished from other aspects of the real world.[4]
An entity is a thing that exists either physically or logically. An entity may be a physical object such as a house or a car (they exist physically), an event such as a house sale or a car service, or a concept such as a customer transaction or order (they exist logically—as a concept). Although the term entity is the one most commonly used, following Chen we should really distinguish between an entity and an entity-type. An entity-type is a category. An entity, strictly speaking, is an instance of a given entity-type. There are usually many instances of an entity-type. Because the term entity-type is somewhat cumbersome, most people tend to use the term entity as a synonym for this term
Entities can be thought of as nouns. Examples: a computer, an employee, a song, a mathematical theorem, etc.
A relationship captures how entities are related to one another. Relationships can be thought of as verbs, linking two or more nouns. Examples: an owns relationship between a company and a computer, a supervises relationship between an employee and a department, a performs relationship between an artist and a song, a proves relationship between a mathematician and a conjecture, etc.
The model’s linguistic aspect described above is utilized in the declarative database query language ERROL, which mimics natural language constructs. ERROL’s semantics and implementation are based on reshaped relational algebra (RRA), a relational algebra that is adapted to the entity–relationship model and captures its linguistic aspect.
Entities and relationships can both have attributes. Examples: an employee entity might have a Social Security Number (SSN) attribute, while a proved relationship may have a date attribute.
All entities except weak entities must have a minimal set of uniquely identifying attributes which may be used as a unique/primary key.
Entity–relationship diagrams don’t show single entities or single instances of relations. Rather, they show entity sets (all entities of the same entity type) and relationship sets (all relationships of the same relationship type). Examples: a particular song is an entity; the collection of all songs in a database is an entity set; the eaten relationship between a child and his lunch is a single relationship; the set of all such child-lunch relationships in a database is a relationship set. In other words, a relationship set corresponds to a relation in mathematics, while a relationship corresponds to a member of the relation.
Certain cardinality constraints on relationship sets may be indicated as well.
Mapping natural language[edit]
Chen proposed the following “rules of thumb” for mapping natural language descriptions into ER diagrams: “English, Chinese and ER diagrams” by Peter Chen.
Physical view show how data is actually stored.
Relationships, roles and cardinalities
In Chen’s original paper he gives an example of a relationship and its roles. He describes a relationship “marriage” and its two roles “husband” and “wife”.
A person plays the role of husband in a marriage (relationship) and another person plays the role of wife in the (same) marriage. These words are nouns. That is no surprise; naming things requires a noun.
Chen’s terminology has also been applied to earlier ideas. The lines, arrows and crow’s-feet of some diagrams owes more to the earlier Bachman diagrams than to Chen’s relationship diagrams.
Another common extension to Chen’s model is to “name” relationships and roles as verbs or phrases.
Role naming
It has also become prevalent to name roles with phrases such as is the owner of and is owned by. Correct nouns in this case are owner and possession. Thus person plays the role of owner and car plays the role of possession rather than person plays the role of, is the owner of, etc.
The use of nouns has direct benefit when generating physical implementations from semantic models. When a person has two relationships with car then it is possible to generate names such as owner_person and driver_person, which are immediately meaningful.[5]
Cardinalities
Modifications to the original specification can be beneficial. Chen described look-across cardinalities. As an aside, the Barker–Ellis notation, used in Oracle Designer, uses same-side for minimum cardinality (analogous to optionality) and role, but look-across for maximum cardinality (the crows foot).[clarification needed]
In Merise,[6] Elmasri & Navathe[7] and others[8] there is a preference for same-side for roles and both minimum and maximum cardinalities. Recent researchers (Feinerer,[9] Dullea et al.[10]) have shown that this is more coherent when applied to n-ary relationships of order greater than 2.
In Dullea et al. one reads “A ‘look across’ notation such as used in the UML does not effectively represent the semantics of participation constraints imposed on relationships where the degree is higher than binary.”
In Feinerer it says “Problems arise if we operate under the look-across semantics as used for UML associations. Hartmann[11] investigates this situation and shows how and why different transformations fail.” (Although the “reduction” mentioned is spurious as the two diagrams 3.4 and 3.5 are in fact the same) and also “As we will see on the next few pages, the look-across interpretation introduces several difficulties that prevent the extension of simple mechanisms from binary to n-ary associations.”

Various methods of representing the same one to many relationship. In each case, the diagram shows the relationship between a person and a place of birth: each person must have been born at one, and only one, location, but each location may have had zero or more people born at it.

Two related entities shown using Crow’s Foot notation. In this example, an optional relationship is shown between Artist and Song; the symbols closest to the song entity represents “zero, one, or many”, whereas a song has “one and only one” Artist. The former is therefore read as, an Artist (can) perform(s) “zero, one, or many” song(s).
Chen’s notation for entity–relationship modeling uses rectangles to represent entity sets, and diamonds to represent relationships appropriate for first-class objects: they can have attributes and relationships of their own. If an entity set participates in a relationship set, they are connected with a line.
Attributes are drawn as ovals and are connected with a line to exactly one entity or relationship set.
Cardinality constraints are expressed as follows:
- a double line indicates a participation constraint, totality or surjectivity: all entities in the entity set must participate in at least one relationship in the relationship set;
- an arrow from entity set to relationship set indicates a key constraint, i.e. injectivity: each entity of the entity set can participate in at most one relationship in the relationship set;
- a thick line indicates both, i.e. bijectivity: each entity in the entity set is involved in exactly one relationship.
- an underlined name of an attribute indicates that it is a key: two different entities or relationships with this attribute always have different values for this attribute.
Attributes are often omitted as they can clutter up a diagram; other diagram techniques often list entity attributes within the rectangles drawn for entity sets.
Related diagramming convention techniques:
Crow’s foot notation, the beginning of which dates back to an article by Gordon Everest (1976),[12] is used in Barker’s notation, Structured Systems Analysis and Design Method (SSADM) and information technology engineering. Crow’s foot diagrams represent entities as boxes, and relationships as lines between the boxes. Different shapes at the ends of these lines represent the relative cardinality of the relationship.
Crow’s foot notation was used in the consultancy practice CACI. Many of the consultants at CACI (including Richard Barker) subsequently moved to Oracle UK, where they developed the early versions of Oracle’s CASE tools, introducing the notation to a wider audience.
With this notation, relationships cannot have attributes. Where necessary, relationships are promoted to entities in their own right: for example, if it is necessary to capture where and when an artist performed a song, a new entity “performance” is introduced (with attributes reflecting the time and place), and the relationship of an artist to a song becomes an indirect relationship via the performance (artist-performs-performance, performance-features-song).
Three symbols are used to represent cardinality:
- the ring represents “zero”
- the dash represents “one”
- the crow’s foot represents “many” or “infinite”
These symbols are used in pairs to represent the four types of cardinality that an entity may have in a relationship. The inner component of the notation represents the minimum, and the outer component represents the maximum.
- ring and dash → minimum zero, maximum one (optional)
- dash and dash → minimum one, maximum one (mandatory)
- ring and crow’s foot → minimum zero, maximum many (optional)
- dash and crow’s foot → minimum one, maximum many (mandatory)
Model usability issues
![[icon]](https://upload.wikimedia.org/wikipedia/commons/thumb/1/1c/Wiki_letter_w_cropped.svg/20px-Wiki_letter_w_cropped.svg.png) | This section needs expansion with: fan trap causes. You can help by adding to it. (February 2018) |
In using a modeled database, users can encounter two well known issues where the returned results mean something other than the results assumed by the query author.
The first is the ‘fan trap’. It occurs with a (master) table that links to multiple tables in a one-to-many relationship. The issue derives its name from the way the model looks when it’s drawn in an entity–relationship diagram: the linked tables ‘fan out’ from the master table. This type of model looks similar to a star schema, a type of model used in data warehouses. When trying to calculate sums over aggregates using standard SQL over the master table, unexpected (and incorrect) results may occur. The solution is to either adjust the model or the SQL. This issue occurs mostly in databases for decision support systems, and software that queries such systems sometimes includes specific methods for handling this issue.
The second issue is a ‘chasm trap’. A chasm trap occurs when a model suggests the existence of a relationship between entity types, but the pathway does not exist between certain entity occurrences. For example, a Building has one-or-more Rooms, that hold zero-or-more Computers. One would expect to be able to query the model to see all the Computers in the Building. However, Computers not currently assigned to a Room (because they are under repair or somewhere else) are not shown on the list. Another relation between Building and Computers is needed to capture all the computers in the building. This last modelling issue is the result of a failure to capture all the relationships that exist in the real world in the model. See Entity-Relationship Modelling 2 for details.
Entity–relationships and semantic modeling
Semantic model
A semantic model is a model of concepts, it is sometimes called a “platform independent model”. It is an intensional model. At least since Carnap, it is well known that:[13]
- “…the full meaning of a concept is constituted by two aspects, its intension and its extension. The first part comprises the embedding of a concept in the world of concepts as a whole, i.e. the totality of all relations to other concepts. The second part establishes the referential meaning of the concept, i.e. its counterpart in the real or in a possible world”.
Extension model
An extensional model is one that maps to the elements of a particular methodology or technology, and is thus a “platform specific model”. The UML specification explicitly states that associations in class models are extensional and this is in fact self-evident by considering the extensive array of additional “adornments” provided by the specification over and above those provided by any of the prior candidate “semantic modelling languages”.“UML as a Data Modeling Notation, Part 2”
Entity–relationship origins
Peter Chen, the father of ER modeling said in his seminal paper:
- “The entity-relationship model adopts the more natural view that the real world consists of entities and relationships. It incorporates some of the important semantic information about the real world.” [1]
In his original 1976 article Chen explicitly contrasts entity–relationship diagrams with record modelling techniques:
- “The data structure diagram is a representation of the organization of records and is not an exact representation of entities and relationships.“
Several other authors also support Chen’s program:[14] [15] [16] [17] [18]
Philosophical alignment
Chen is in accord with philosophical traditions from the time of the Ancient Greek philosophers: Plato and Aristotle.[19] Plato himself associates knowledge with the apprehension of unchanging Forms (namely, archetypes or abstract representations of the many types of things, and properties) and their relationships to one another.
Limitations
- An ER model is primarily conceptual, an ontology that expresses predicates in a domain of knowledge.
- ER models are readily used to represent relational database structures (after Codd and Date) but not so often to represent other kinds of data structure (data warehouses, document stores etc.)
- Some ER model notations include symbols to show super-sub-type relationships and mutual exclusion between relationships; some don’t.
- An ER model does not show an entity’s life history (how its attributes and/or relationships change over time in response to events). For many systems, such state changes are nontrivial and important enough to warrant explicit specification.
- Some[who?] have extended ER modeling with constructs to represent state changes, an approach supported by the original author;[20] an example is Anchor Modeling.
- Others model state changes separately, using state transition diagrams or some other process modeling technique.
- Many other kinds of diagram are drawn to model other aspects of systems, including the 14 diagram types offered by UML.[21]
- Today, even where ER modeling could be useful, it is uncommon because many use tools that support similar kinds of model, notably class diagrams for OO programming and data models for relational database management systems. Some of these tools can generate code from diagrams and reverse-engineer diagrams from code.
- In a survey, Brodie and Liu[22] could not find a single instance of entity–relationship modeling inside a sample of ten Fortune 100 companies. Badia and Lemire[23] blame this lack of use on the lack of guidance but also on the lack of benefits, such as lack of support for data integration.
- The enhanced entity–relationship model (EER modeling) introduces several concepts not in ER modeling, but are closely related to object-oriented design, like is-a relationships.
- For modelling temporal databases, numerous ER extensions have been considered.[24] Similarly, the ER model was found unsuitable for multidimensional databases (used in OLAP applications); no dominant conceptual model has emerged in this field yet, although they generally revolve around the concept of OLAP cube (also known as data cube within the field).[25]
See also
References
- ^ Jump up to:a b Chen, Peter (March 1976). “The Entity-Relationship Model – Toward a Unified View of Data”. ACM Transactions on Database Systems. 1 (1): 9–36. CiteSeerX 10.1.1.523.6679. doi:10.1145/320434.320440. S2CID 52801746.
- ^ A.P.G. Brown, “Modelling a Real-World System and Designing a Schema to Represent It”, in Douque and Nijssen (eds.), Data Base Description, North-Holland, 1975, ISBN 0-7204-2833-5.
- ^ “Lesson 5: Supertypes and Subtypes”. docs.microsoft.com.
- ^ Beynon-Davies, Paul (2004). Database Systems. Basingstoke, UK: Palgrave: Houndmills. ISBN 978-1403916013.
- ^ “The Pangrammaticon: Emotion and Society”. January 3, 2013.
- ^ Hubert Tardieu, Arnold Rochfeld and René Colletti La methode MERISE: Principes et outils (Paperback – 1983)
- ^ Elmasri, Ramez, B. Shamkant, Navathe, Fundamentals of Database Systems, third ed., Addison-Wesley, Menlo Park, CA, USA, 2000.
- ^ ER 2004 : 23rd International Conference on Conceptual Modeling, Shanghai, China, November 8-12, 2004. 2004-10-27. ISBN 9783540237235.
- ^ “A Formal Treatment of UML Class Diagrams as an Efficient Method for Configuration Management 2007” (PDF).
- ^ “James Dullea, Il-Yeol Song, Ioanna Lamprou – An analysis of structural validity in entity-relationship modeling 2002” (PDF).
- ^ Hartmann, Sven. “Reasoning about participation constraints and Chen’s constraints Archived 2013-05-10 at the Wayback Machine“. Proceedings of the 14th Australasian database conference-Volume 17. Australian Computer Society, Inc., 2003.
- ^ G. Everest, “BASIC DATA STRUCTURE MODELS EXPLAINED WITH A COMMON EXAMPLE”, in Computing Systems 1976, Proceedings Fifth Texas Conference on Computing Systems, Austin,TX, 1976 October 18–19, pages 39-46. (Long Beach, CA: IEEE Computer Society Publications Office).
- ^ “The Role of Intensional and Extensional Interpretation in Semantic Representations”.
- ^ Kent in “Data and Reality” :
- “One thing we ought to have clear in our minds at the outset of a modelling endeavour is whether we are intent on describing a portion of “reality” (some human enterprise) or a data processing activity.”
- ^ Abrial in “Data Semantics” : “… the so called “logical” definition and manipulation of data are still influenced (sometimes unconsciously) by the “physical” storage and retrieval mechanisms currently available on computer systems.”
- ^ Stamper: “They pretend to describe entity types, but the vocabulary is from data processing: fields, data items, values. Naming rules don’t reflect the conventions we use for naming people and things; they reflect instead techniques for locating records in files.”
- ^ In Jackson’s words: “The developer begins by creating a model of the reality with which the system is concerned, the reality that furnishes its [the system’s] subject matter …”
- ^ Elmasri, Navathe: “The ER model concepts are designed to be closer to the user’s perception of data and are not meant to describe the way in which data will be stored in the computer.”
- ^ Paolo Rocchi, Janus-Faced Probability, Springer, 2014, p. 62.
- ^ P. Chen. Suggested research directions for a new frontier: Active conceptual modeling. ER 2006, volume 4215 of Lecture Notes in Computer Science, pages 1–4. Springer Berlin / Heidelberg, 2006.
- ^ Carte, Traci A.; Jasperson, Jon (Sean); and Cornelius, Mark E. (2020) “Integrating ERD and UML Concepts When Teaching Data Modeling,” Journal of Information Systems Education: Vol. 17 : Iss. 1 , Article 9.
- ^ The power and limits of relational technology in the age of information ecosystems Archived 2016-09-17 at the Wayback Machine. On The Move Federated Conferences, 2010.
- ^ A. Badia and D. Lemire. A call to arms: revisiting database design. Citeseerx,
- ^ Gregersen, Heidi; Jensen, Christian S. (1999). “Temporal Entity-Relationship models—a survey”. IEEE Transactions on Knowledge and Data Engineering. 11 (3): 464–497. CiteSeerX 10.1.1.1.2497. doi:10.1109/69.774104.
- ^ RICCARDO TORLONE (2003). “Conceptual Multidimensional Models” (PDF). In Maurizio Rafanelli (ed.). Multidimensional Databases: Problems and Solutions. Idea Group Inc (IGI). ISBN 978-1-59140-053-0.
Further reading
- Chen, Peter (2002). “Entity-Relationship Modeling: Historical Events, Future Trends, and Lessons Learned” (PDF). Software pioneers. Springer-Verlag. pp. 296–310. ISBN 978-3-540-43081-0.
- Barker, Richard (1990). CASE Method: Entity Relationship Modelling. Addison-Wesley. ISBN 978-0201416961.
- Barker, Richard (1990). CASE Method: Tasks and Deliverables. Addison-Wesley. ISBN 978-0201416978.
- Mannila, Heikki; Räihä, Kari-Jouko (1992). The Design of Relational Databases. Addison-Wesley. ISBN 978-0201565232.
- Thalheim, Bernhard (2000). Entity-Relationship Modeling: Foundations of Database Technology. Springer. ISBN 978-3-540-65470-4.
- Bagui, Sikha; Earp, Richard (2011). Database Design Using Entity-Relationship Diagrams (2nd ed.). CRC Press. ISBN 978-1-4398-6176-9.
External links