What is facial recognition?
Facial recognition is a way of identifying or confirming an individual’s identity using their face. Facial recognition systems can be used to identify people in photos, videos, or in real-time.
Facial recognition is a category of biometric security. Other forms of biometric software include voice recognition, fingerprint recognition, and eye retina or iris recognition. The technology is mostly used for security and law enforcement, though there is increasing interest in other areas of use.
How does facial recognition work?
Many people are familiar with face recognition technology through the FaceID used to unlock iPhones (however, this is only one application of face recognition). Typically, facial recognition does not rely on a massive database of photos to determine an individual’s identity — it simply identifies and recognizes one person as the sole owner of the device, while limiting access to others.
Beyond unlocking phones, facial recognition works by matching the faces of people walking past special cameras, to images of people on a watch list. The watch lists can contain pictures of anyone, including people who are not suspected of any wrongdoing, and the images can come from anywhere — even from our social media accounts. Facial technology systems can vary, but in general, they tend to operate as follows:
Step 1: Face detection
The camera detects and locates the image of a face, either alone or in a crowd. The image may show the person looking straight ahead or in profile.
Step 2: Face analysis
Next, an image of the face is captured and analyzed. Most facial recognition technology relies on 2D rather than 3D images because it can more conveniently match a 2D image with public photos or those in a database. The software reads the geometry of your face. Key factors include the distance between your eyes, the depth of your eye sockets, the distance from forehead to chin, the shape of your cheekbones, and the contour of the lips, ears, and chin. The aim is to identify the facial landmarks that are key to distinguishing your face.
Step 3: Converting the image to data
The face capture process transforms analog information (a face) into a set of digital information (data) based on the person’s facial features. Your face’s analysis is essentially turned into a mathematical formula. The numerical code is called a faceprint. In the same way that thumbprints are unique, each person has their own faceprint.
Step 4: Finding a match
Your faceprint is then compared against a database of other known faces. For example, the FBI has access to up to 650 million photos, drawn from various state databases. On Facebook, any photo tagged with a person’s name becomes a part of Facebook’s database, which may also be used for facial recognition. If your faceprint matches an image in a facial recognition database, then a determination is made.
Of all the biometric measurements, facial recognition is considered the most natural. Intuitively, this makes sense, since we typically recognize ourselves and others by looking at faces, rather than thumbprints and irises. It is estimated that over half of the world’s population is touched by facial recognition technology regularly.
How facial recognition is used
The technology is used for a variety of purposes. These include:
Unlocking phones
Various phones, including the most recent iPhones, use face recognition to unlock the device. The technology offers a powerful way to protect personal data and ensures that sensitive data remains inaccessible if the phone is stolen. Apple claims that the chance of a random face unlocking your phone is about one in 1 million.
Law enforcement
Facial recognition is regularly being used by law enforcement. According to this NBC report, the technology is increasing amongst law enforcement agencies within the US, and the same is true in other countries. Police collects mugshots from arrestees and compare them against local, state, and federal face recognition databases. Once an arrestee’s photo has been taken, their picture will be added to databases to be scanned whenever police carry out another criminal search.
Also, mobile face recognition allows officers to use smartphones, tablets, or other portable devices to take a photo of a driver or a pedestrian in the field and immediately compare that photo against to one or more face recognition databases to attempt an identification.
Airports and border control
Facial recognition has become a familiar sight at many airports around the world. Increasing numbers of travellers hold biometric passports, which allow them to skip the ordinarily long lines and instead walk through an automated ePassport control to reach the gate faster. Facial recognition not only reduces waiting times but also allows airports to improve security. The US Department of Homeland Security predicts that facial recognition will be used on 97% of travellers by 2023. As well as at airports and border crossings, the technology is used to enhance security at large-scale events such as the Olympics.
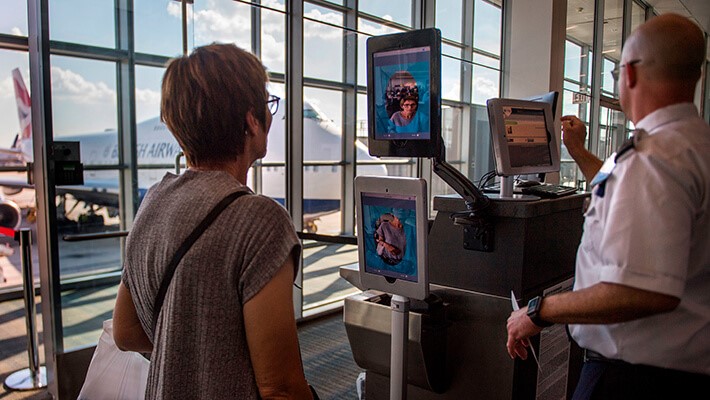
Finding missing persons
Facial recognition can be used to find missing persons and victims of human trafficking. Suppose missing individuals are added to a database. In that case, law enforcement can be alerted as soon as they are recognized by face recognition — whether it is in an airport, retail store, or other public space.
Reducing retail crime
Facial recognition is used to identify when known shoplifters, organized retail criminals, or people with a history of fraud enter stores. Photographs of individuals can be matched against large databases of criminals so that loss prevention and retail security professionals can be notified when shoppers who potentially represent a threat enter the store.
Improving retail experiences
The technology offers the potential to improve retail experiences for customers. For example, kiosks in stores could recognize customers, make product suggestions based on their purchase history, and point them in the right direction. “Face pay” technology could allow shoppers to skip long checkout lines with slower payment methods.
Banking
Biometric online banking is another benefit of face recognition. Instead of using one-time passwords, customers can authorize transactions by looking at their smartphone or computer. With facial recognition, there are no passwords for hackers to compromise. If hackers steal your photo database, ‘liveless’ detection – a technique used to determine whether the source of a biometric sample is a live human being or a fake representation – should (in theory) prevent them from using it for impersonation purposes. Face recognition could make debit cards and signatures a thing of the past.
Marketing and advertising
Marketers have used facial recognition to enhance consumer experiences. For example, frozen pizza brand DiGiorno used facial recognition for a 2017 marketing campaign where it analyzed the expressions of people at DiGiorno-themed parties to gauge people’s emotional reactions to pizza. Media companies also use facial recognition to test audience reaction to movie trailers, characters in TV pilots, and optimal placement of TV promotions. Billboards that incorporate face recognition technology – such as London’s Piccadilly Circus – means brands can trigger tailored advertisements.
Healthcare
Hospitals use facial recognition to help with patient care. Healthcare providers are testing the use of facial recognition to access patient records, streamline patient registration, detect emotion and pain in patients, and even help to identify specific genetic diseases. AiCure has developed an app that uses facial recognition to ensure that people take their medication as prescribed. As biometric technology becomes less expensive, adoption within the healthcare sector is expected to increase.
Tracking student or worker attendance
Some educational institutions in China use face recognition to ensure students are not skipping class. Tablets are used to scan students’ faces and match them to photos in a database to validate their identities. More broadly, the technology can be used for workers to sign in and out of their workplaces, so that employers can track attendance.
Recognizing drivers
According to this consumer report, car companies are experimenting with facial recognition to replace car keys. The technology would replace the key to access and start the car and remember drivers’ preferences for seat and mirror positions and radio station presets.
Monitoring gambling addictions
Facial recognition can help gambling companies protect their customers to a higher degree. Monitoring those entering and moving around gambling areas is difficult for human staff, especially in large crowded spaces such as casinos. Facial recognition technology enables companies to identify those who are registered as gambling addicts and keeps a record of their play so staff can advise when it is time to stop. Casinos can face hefty fines if gamblers on voluntary exclusion lists are caught gambling.
Examples of facial recognition technology
- Amazon previously promoted its cloud-based face recognition service named Rekognition to law enforcement agencies. However, in a June 2020 blog post, the company announced it was planning a one-year moratorium on the use of its technology by police. The rationale for this was to allow time for US federal laws to be initiated, to protect human rights and civil liberties.
- Apple uses facial recognition to help users quickly unlock their phones, log in to apps, and make purchases.
- British Airways enables facial recognition for passengers boarding flights from the US. Travellers’ faces can be scanned by a camera to have their identity verified to board their plane without showing their passport or boarding pass. The airline has been using the technology on UK domestic flights from Heathrow and is working towards biometric boarding on international flights from the airport.
- Cigna, a US-based healthcare insurer, allows customers in China to file health insurance claims which are signed using a photo, rather than a written signature, in a bid to cut down on instances of fraud.
- Coca-Cola has used facial recognition in several ways across the world. Examples include rewarding customers for recycling at some of its vending machines in China, delivering personalized ads on its vending machines in Australia, and for event marketing in Israel.
- Facebook began using facial recognition in the US in 2010 when it automatically tagged people in photos using its tag suggestions tool. The tool scans a user’s face and offers suggestions about who that person is. Since 2019, Facebook has made the feature opt-in as part of a drive to become more privacy focused. Facebook provides information on how you can opt-in or out of face recognition here.
- Google incorporates the technology into Google Photos and uses it to sort pictures and automatically tag them based on the people recognized.
- MAC make-up, uses facial recognition technology in some of its brick-and-mortar stores, allowing customers to virtually “try on” make-up using in-store augmented reality mirrors.
- McDonald’s has used facial recognition in its Japanese restaurants to assess the quality of customer service provided there, including analyzing whether its employees are smiling while assisting customers.
- Snapchat is one of the pioneers of facial recognition software: it allows brands and organizations to create filters which mould to the user’s face — hence the ubiquitous puppy dog faces and flower crown filters seen on social media.
Technology companies that provide facial recognition technology include:
- Kairos
- Noldus
- Affectiva
- Sightcorp
- Nviso
Advantages of face recognition
Aside from unlocking your smartphone, facial recognition brings other benefits:
Increased security
On a governmental level, facial recognition can help to identify terrorists or other criminals. On a personal level, facial recognition can be used as a security tool for locking personal devices and for personal surveillance cameras.
Reduced crime
Face recognition makes it easier to track down burglars, thieves, and trespassers. The sole knowledge of the presence of a face recognition system can serve as a deterrence, especially to petty crime. Aside from physical security, there are benefits to cybersecurity as well. Companies can use face recognition technology as a substitute for passwords to access computers. In theory, the technology cannot be hacked as there is nothing to steal or change, as is the case with a password.
Removing bias from stop and search
Public concern over unjustified stops and searches is a source of controversy for the police — facial recognition technology could improve the process. By singling out suspects among crowds through an automated rather than human process, face recognition technology could help reduce potential bias and decrease stops and searches on law-abiding citizens.
Greater convenience
As the technology becomes more widespread, customers will be able to pay in stores using their face, rather than pulling out their credit cards or cash. This could save time in checkout lines. Since there is no contact required for facial recognition as there is with fingerprinting or other security measures – useful in the post-COVID world – facial recognition offers a quick, automatic, and seamless verification experience.
Faster processing
The process of recognizing a face takes only a second, which has benefits for the companies that use facial recognition. In an era of cyber-attacks and advanced hacking tools, companies need both secure and fast technologies. Facial recognition enables quick and efficient verification of a person’s identity.
Integration with other technologies
Most facial recognition solutions are compatible with most security software. In fact, it is easily integrated. This limits the amount of additional investment required to implement it.
Disadvantages of face recognition
While some people do not mind being filmed in public and do not object to the use of facial recognition where there is a clear benefit or rationale, the technology can inspire intense reactions from others. Some of the disadvantages or concerns include:
Surveillance
Some worry that the use of facial recognition along with ubiquitous video cameras, artificial intelligence, and data analytics creates the potential for mass surveillance, which could restrict individual freedom. While facial recognition technology allows governments to track down criminals, it could also allow them to track down ordinary and innocent people at any time.
Scope for error
Facial recognition data is not free from error, which could lead to people being implicated for crimes they have not committed. For example, a slight change in camera angle or a change in appearance, such as a new hairstyle, could lead to error. In 2018, Newsweek reported that Amazon’s facial recognition technology had falsely identified 28 members of the US Congress as people arrested for crimes.
Breach of privacy
The question of ethics and privacy is the most contentious one. Governments have been known to store several citizens’ pictures without their consent. In 2020, the European Commission said it was considering a ban on facial recognition technology in public spaces for up to five years, to allow time to work out a regulatory framework to prevent privacy and ethical abuses.
Massive data storage
Facial recognition software relies on machine learning technology, which requires massive data sets to “learn” to deliver accurate results. Such large data sets require robust data storage. Small and medium-sized companies may not have sufficient resources to store the required data.
Facial recognition security – how to protect yourself
While biometric data is generally considered one of the most reliable authentication methods, it also carries significant risk. That’s because if someone’s credit card details are hacked, that person has the option to freeze their credit and take steps to change the personal information that was breached. What do you do if you lose your digital ‘face’?
Around the world, biometric information is being captured, stored, and analyzed in increasing quantities, often by organizations and governments, with a mixed record on cybersecurity. A question increasingly being asked is, how safe is the infrastructure that holds and processes all this data?
As facial recognition software is still in its relative infancy, the laws governing this area are evolving (and sometimes non-existent). Regular citizens whose information is compromised have relatively few legal avenues to pursue. Cybercriminals often elude the authorities or are sentenced years after the fact, while their victims receive no compensation and are left to fend for themselves.
As the use of facial recognition becomes more widespread, the scope for hackers to steal your facial data to commit fraud — increases.
Biometric technology offers very compelling security solutions. Despite the risks, the systems are convenient and hard to duplicate. These systems will continue to develop in the future — the challenge will be to maximize their benefits while minimizing their risks.
Source