The application is simple enough, but the technology behind it could be sophisticated. The magic that makes the aforementioned scenario possible is natural language processing (NLP). NLP is far more than a pillar for building Siri. It can also empower many other AI-infused applications in the real world.
This article first explains what NLP is and later moves on to introduce five real-world applications of NLP.
What is NLP?
From chatbots to Siri, from virtual support agents to knowledge graphs, the application and usage of NLP are ubiquitous in our daily life. NLP stands for “Natural Language Processing”. Simply put, NLP is the ability of a machine to understand human language. It is the bridge that enables humans to directly interact and communicate with machines. NLP is a subfield of artificial intelligence (AI) and in Bill Gates’s words, “NLP is the pearl in the crown of AI.”
With the ever-expanding market size of NLP, countless companies are investing heavily in this industry, and their product lines vary. Many different but specific systems for various tasks and needs can be built by leveraging the power of NLP.
The Five Real World NLP Applications
The most popular exciting and flourishing real-world applications of NLP include: Conversational user interface, AI-powered call quality assessment, Intelligent outbound calls, AI-powered call operators, and knowledge graphs, to name a few.
Chatbots in E-commerce
Over five years ago, Amazon already realized the potential benefit of applying NLP to their customer service channels. Back then, when customers had issues with their product orderings, the only way they could resort was by calling the customer service agents. However, what they could get from the other side of the phone was “Your call is important to us. Please hold, we’re currently experiencing a high call load. “ most of the time. Thankfully, Amazon immediately realized the damaging effect this could have on their brand image and tried to build chatbots.
Nowadays, when you want to quickly get, for example, a refund online, there’s a much more convenient way! All you need to do is to activate the Amazon customer service chatbot and type in your ordering information and make a refund request. The chatbot interacts and replies the same way a real human does. Apart from the chatbots that deal with post-sales customer experience, chatbots also offer pre-sales consulting. If you have any questions about the product you are going to buy, you can simply chat with a bot and get the answers.
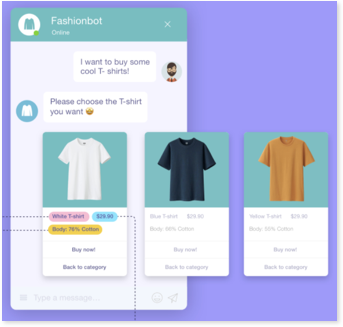
With the emergence of new concepts like metaverse, NLP can do more than power AI chatbots. Avatars for customer support in the metaverse rely on the NLP technology. Giving customers more realistic chatting experiences.
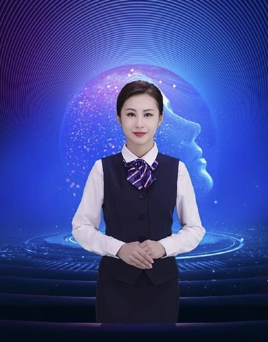
Conversational User Interface
Another more trendy and promising application is interactive systems. Many well-recognized companies are betting big on CUI ( Conversational user interface). CUI is the general term to describe those user interfaces for computers that can simulate conversations with real human beings.
The most common CUIs in our everyday life are Apple’s Siri, Microsoft’s Cortana, Google’s Google Assistant, Amazon’s Alexa, etc.
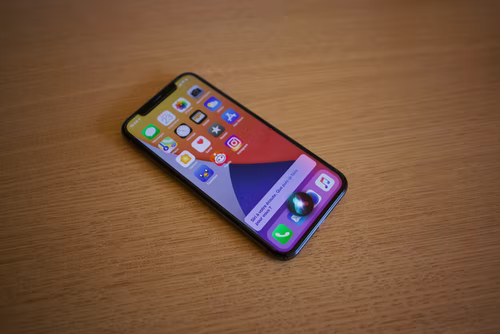
In addition, CUIs can also be embedded into cars, especially EVs (electric vehicles). NIO, an automobile manufacturer dedicated to designing and developing EVs, launched its own set of CUI named NOMI in 2018. Visually, the CUIs in cars can work in the same way as Siri. Drivers can focus on steering the car while asking the CUI to adjust A/C temperature, play a song, lock windows/doors, navigate drivers to the nearest gas station, etc.
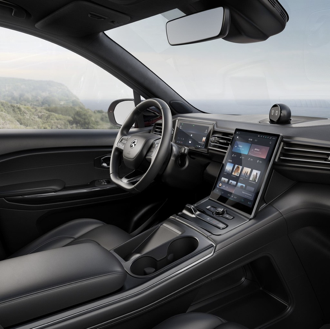
The Algorithm Behind
Despite all the fancy algorithms the technical media have boasted about, one of the most fundamental ways to build a chatbot is to construct and organize FAQ pairs(or more straightforwardly, question-answer pairs) and use NLP algorithms to figure out if the user query matches anyone of your FAQ knowledge base. A simple FAQ example would be like this:
Q: Can I have some coffee?
A: No, I’d rather have some ribs.
Now that this FAQ pair is already stored in your NLP system, the user can now simply ask a similar question for example: “coffee, please!”. If your algorithm is smart enough, it will figure out that “coffee, please” has a great resemblance to “Can I have some coffee?” and will output the corresponding answer “No, I’d rather have some ribs.” And that’s how things are done.
For a very long time, FAQ search algorithms are solely based on inverted indexing. In this case, you first do tokenization on the original sentence and put tokens and documents into systems like ElasticSearch, which uses inverted-index for indexing and algorithms like TF-IDF or BM25 for scoring.
This algorithm works just as fine until the deep learning era arrives. One of the most substantial problems with the algorithm above is that neither tokenization nor inverted indexing takes into account the semantics of the sentences. For instance, in the example above, users could say “ Can I have a cup of Cappuccino” instead. Now with tokenization and inverted-indexing, there’s a very big chance that the system won’t recognize “coffee” and “a cup of Cappuccino” as the same thing and would thus fail to understand the sentence. AI engineers have to do a lot of workarounds for these kinds of issues.
But things got much better with deep learning. With pre-trained models like BERT and pipelines like Towhee, we can easily encode all sentences into vectors and store them in a vector database, for example, Milvus, and simply calculate vector distance to figure out the semantic resembles of sentences.
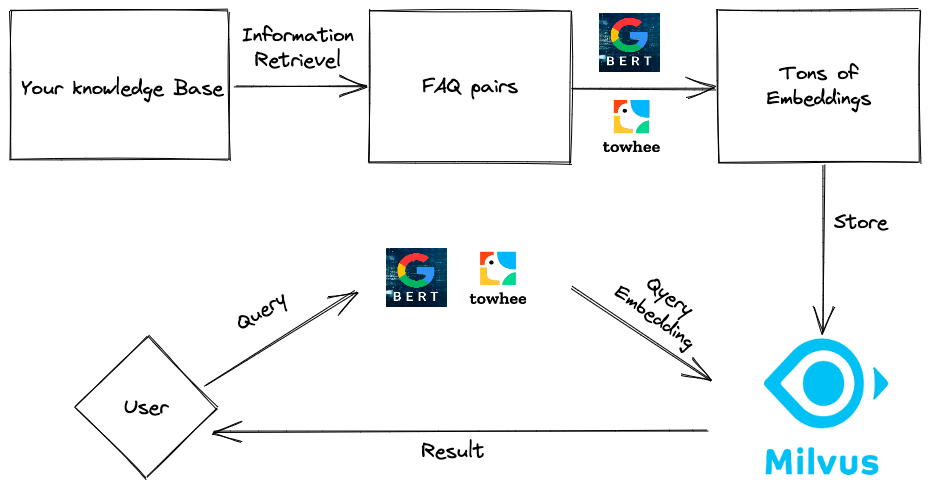
AI-powered Call Quality Control
Call centers are indispensable for many large companies that care about customer experience. To better spot issues and improve call quality, assessment is necessary. However, the problem is that call centers of large multi-national companies receive tremendous amounts of inbound calls per day. Therefore, it is impractical to listen to each of the millions of calls and make the evaluation. Most of the time, when you hear “in order to improve our service, this call could be recorded.” from the other end of the phone, it doesn’t necessarily mean your call would be checked for quality of service. In fact, even in big organizations, only 2%-3% of the calls would be replayed and checked manually by quality control people.
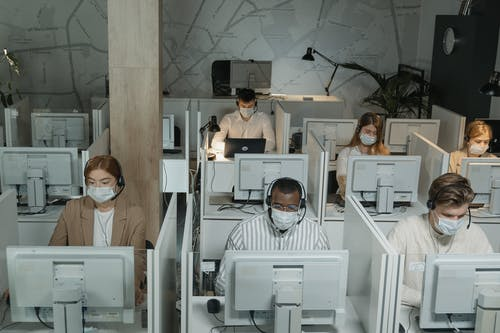
This is where NLP can help. An AI-powered call quality control engine powered by NLP can automatically spot the issues incalls and can handle massive volumes of calls in a relatively short period of time. The engine helps detect if the call operator uses the proper opening and ending sentences, and avoids that banned slang and taboo words in the call. This would easily increase the check rate from 2%-3% to 100%, with even less manpower and other costs.
With a typical AI-powered call quality control service, users need to first upload the call recordings to the service. Then the technology of Automatic speech recognition (ASR) is used to transcribe the audio files into texts. All the texts are subsequently vectorized using deep learning models and subsequently stored in a vector database. The service compares the similarity between the text vectors and vectors generated from a certain set of criteria such as taboo word vectors and vectors of desired opening and closing sentences. With efficient vector similarity search, handling great volumes of call recordings can be much more accurate and less time-consuming.
Intelligent outbound calls
Believe it or not, some of the phone calls you receive are not from humans! Chances are that it is a robot talking from the other side of the call. To reduce operation costs, some companies might leverage AI phone calls for marketing purposes and much more. Google launched Google Duplex back in 2018, a system that can conduct human-computer conversations and accomplish real-world tasks over the phone. The mechanism behind AI phone calls is pretty much the same as that behind chatbots.
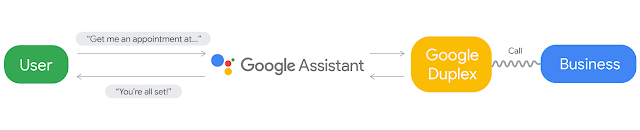
In other cases, you might have also heard something like this on the phone:
“Thank you for calling. To set up a new account, press 1. To modify your password to an existing account, press 2. To speak to our customer service agent, press 0.”,
or in recent years, something like (with a strong robot accent):
“Please tell me what I can help you with. For example, You can ask me ‘check the balance of my account’.”
This is known as interactive voice response (IVR). It is an automated phone system that interacts with callers and performs based on the answers and actions of the callers. The callers are usually offered some choices via a menu. And then their choice will decide how the phone call system acts. If the user request is too complex, the system can route callers to a human agent. This can greatly reduce labor costs and save time for companies.
Intents are usually very helpful when dealing with calls like these. An intent is a group of sentences or dialects representing a certain user intention. For example, “weather forecast” can be intent, and this intent can be triggered with different sentences. See the picture of a Google Dialogflow example below. Intents can be organized together to accomplish complicated interactive human-computer conversations. Like booking a restaurant, ordering a flight ticket, etc.
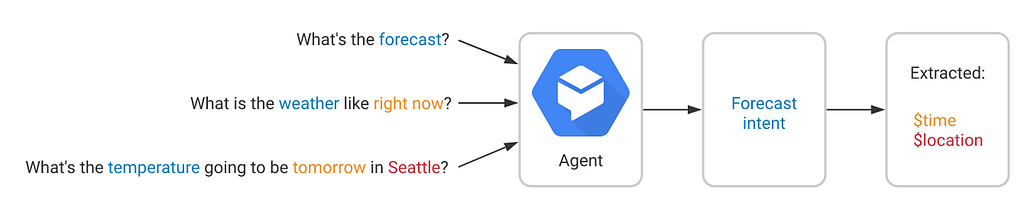
AI-powered call operators
By adopting the technology of NLP, companies can carry call operation services to the next level. Conventionally, call operators need to look up a hundred page-long professional manual to deal with each call from customers and solve each of the user problems case by case. This process is extremely time-consuming and for most of the time cannot satisfy callers with desirable solutions. However, with an AI-powered call center, dealing with customer calls can be both cozy and efficient.
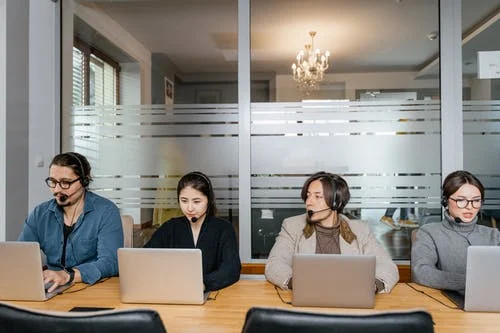
When a customer dials in, the system immediately searches for the customer and their ordering information in the database so that the call operator can have a general idea of the case, like how old the customer is, their marriage status, things they have purchased in the past, etc. During the conversation, the whole chat will be recorded with a live chat log shown on the screen (thanks to living Automatic Speech Recognition). Moreover, when a customer asks a hard question or starts complaining, the machine will catch it automatically, look into the AI database, and tell you what is the best way to respond. With a decent deep learning model, your service could always give your customer >99% correct answers to their questions and can always handle customers’ complaints with the most proper words.
Knowledge graph
A knowledge graph is an information-based graph that consists of nodes, edges, and labels. Where a node (or a vertex) usually represents an entity. It could be a person, a place, an item, or an event. Edges are the lines connecting the nodes. There are also labels that signify the connection or relationship between a pair of nodes. A typical knowledge graph example is shown below:
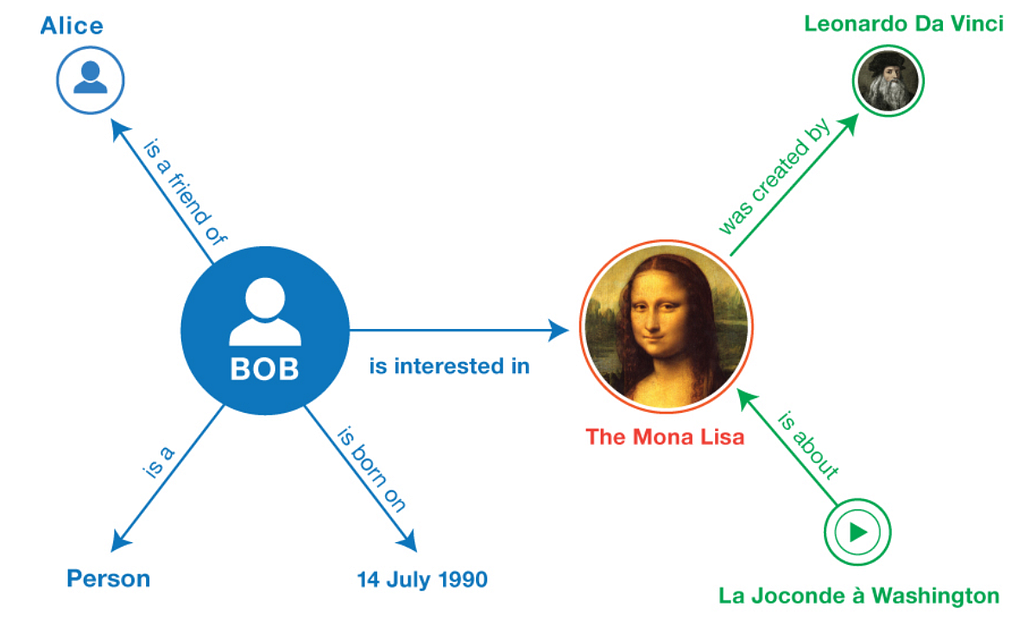
The raw data for constructing a knowledge graph may come from various sources — unstructured docs, semi-structured data, and structured knowledge. Various algorithms must be applied to these data so as to extract entities (nodes) and the relationship between entities (edges). To name a few, one needs to do entity recognition, relations extracting, label mining, entity linking. To build a knowledge graph with data in docs, for instance, we need to first use deep learning pipelines to generate embeddings and store them in a vector database.
Once the knowledge graph is constructed, you can see it as the underlying pillar for many more specific applications like smart search engines, question-answering systems, recommending systems, advertisements, and more.
Endnote
This article introduces the top five real-world NLP applications. Leveraging NLP in your business can greatly reduce operational costs and improve user experience. Of course, apart from the five applications introduced in this article, NLP can facilitate more business scenarios including social media analytics, translation, sentiment analysis, meeting summarizing, and more.
There are also a bunch of NLP+, or more generally, AI+ concepts that are getting more and more popular these few years. For example, with AI + RPA (Robotic process automation). You can easily build smart pipelines that complete workflows automatically for you, such as an expense reimbursement workflow where you just need to upload your receipt, and AI + RPA will do all the rest for you. There’s also AI + OCR, where you just need to take a picture of, say, a contract, and AI will tell you if there’s a mistake in your contract, say, the telephone number of a company doesn’t match the number shown in Google search.